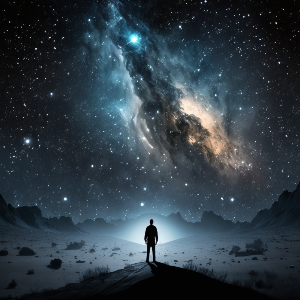
The focus of my last column, titled Crossing the Data Divide: Data Catalogs and the Generative AI Wave, was on the impact of large language models (LLM) and generative artificial intelligence (AI) and how we disseminate knowledge throughout the enterprise and the future role of the data catalogs. Spoiler alert if you have not read it: My position is that they are complementary, but the catalog and its proprietary user interface cease to be what we try to get users to adopt.
In this column, I am looking at the “other end of the stick” — that is, less focus on the platform and more focus on an outcome in the form of AI data assistants and the value they can provide for data leaders.
We are all familiar with automated support agents. They existed long before large language models (LLM) and generative AI. We are also becoming increasingly familiar with creating custom Generative Pre-trained Transformers (GPT) for creating assistants that can be focused on specific areas of expertise to help us answer questions, generate content, summarize complex material, brainstorm ideas, etc. My focus is on that assistant creation capability but for data and analytic-specific purposes.
Long on Expectations and Short of Everything
The primary challenge that all data leaders face is managing the expectations of the C-suite so they have enough breathing room to form and lead all the programs required to apply data and analytics to improve business growth and efficiency. Of course, by breathing room, I mean sufficient budget, people, time, and political support.
The most difficult aspect of managing expectations is educating leaders unfamiliar with the underbelly and complexity of the data world on how much foundational work needs to be done to reach the goal of a highly data-literate employee population with quality data at their fingertips.
We know that it requires a significant investment in multiple programs, including data literacy, data governance, and data cataloging, to name a few. All of which must engage and empower cross-functional technical and business functions.
Unfortunately, even the best at managing expectations rarely have enough budget, people, and C-Suite patience. We need to deliver more, faster, with the resources we have. That’s an impossible task unless we can find a force multiplier.
Staffing up with AI Data Assistants
I provide a framework below for defining AI data assistant capabilities, but first, I want to challenge your perception of the problem and potential solution.
If you agree that it’s unlikely that you will ever have enough budget to staff at the level required, you have little choice but to look toward automation. Not to replace existing positions or substitute human new hires for automation, but because the reality is that there are open requisitions, you will never get.
The second, not-so-subtle, and obvious shift I am challenging you to make is to stop thinking of GPT-based assistants as an application and start thinking of them as human resources that you can add to the team. Think of them as someone with experience and talent who matches your team’s needs.
Job Descriptions and Requirements as a “Hiring” Framework
Once you accept that an AI data assistant can be additional staff, the transition to using traditional job requirements and descriptions is natural.
The goal then becomes thoughtfully composing these as if hiring human resources. This includes thinking about things like level of experience, job families, and how these “hires” will fit in and interact with existing staff. To aid their creation, I suggest you use a simple framework defining the job roles, domain experience, and key benefactors.
Basic Job Roles
I am no human resource expert, but Table 1 below shows how I think about and classify roles.
No. | Role | Description |
1 | Librarians & Curators | Provides assistance with navigating and locating required resources. This includes providing the definition of assets. Maintains collections and classifications of assets. |
2 | Instructors | Teaches “what?” “how?” and “who?” on a wide variety of topics. Creates course curriculum and suggests learning paths. |
3 | Analysts | Provides insights when provided with input, goals, and context. |
4 | Builders | Creates content of various types, which can include technical or business assets. |
5 | Consultants | Provides recommendations based on best practices and situational assessments. Provides reasoning and justification for all recommendations. |
6 | Decision Makers | Makes decisions based on analysis and recommendations provided by others or that they create. Directs others to act on their decisions. |
Table 1: Job Roles
Just like a human, an AI data assistant can play any of these roles; however, it’s well understood that the current state of the art is 1-3 and that capabilities related to 4-6 are still evolving. I would not put blinders on and ignore or exclude those roles. Just think of them as rare talent you will keep your eyes open for, as you do with people.
Domain Experience
Domain experience is self-explanatory. In these areas where you want the new hire to have experience, keep in mind that different job levels (I, II, III) will require an increased depth of experience.
Your list of domains will depend on the current skills you already have staffed and the gaps you want to fill. It will also depend on your future needs, which should be defined in a program roadmap. Table 2 shows a sample list of domains.
Data Governance & Stewardship | Data Cataloging |
Data Quality | Business Analysis & Process Expertise |
Data Privacy & Security | Data Integration |
Descriptive Analytics | Data Architecture |
Diagnostic Analytics | Master Data Management |
Predictive Analytics & Statistics | Prescriptive Analytics & Data Science |
Table 2: Domain Experience
Key Benefactors
This may feel odd, but the idea is to clarify who the role would be serving and what value they would be required to deliver. Said the other way around, it’s understanding the personas for whom the role is being hired.
Understanding the key personas being served when the role is aimed at working cross-functionally is especially important. For instance, working with stewards who are part of business functions, delivering training to the enterprise, or working closely on business process-specific analytics.
The most thorough organizations create persona descriptions of their key internal customers and document their responsibilities, goals, needs, and requirements. This provides clarity and creates a shared understanding of who they are serving.
Bringing It Together
It’s not rocket science, but a proven technique is to use a matrix, as shown in Figure 1 below.
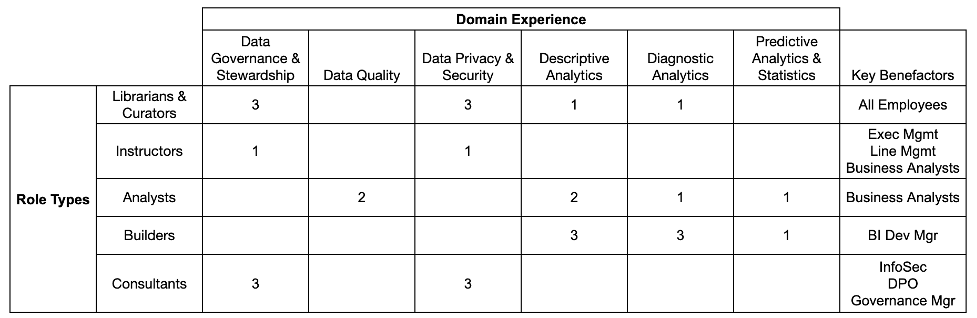
The row headers are the role types, the column headers are the domain experience and a special column for the key benefactor at the end. In the key benefactor column, you would list the persona names.
Instead of just putting a tick mark at the intersection of rows and columns, I prefer to use a number between 1 and 3, representing the level of experience required. Of course, those levels should be documented as part of the domain experience descriptions.
I’ve purposely ignored actual job titles to this point, but naturally, you could also extend this exercise in that direction.
Getting on with the “Hiring”
Once you have the open roles defined you can get on with the “hiring.” But what does that mean in the context of an AI data assistant? It means that you need to do three things:
- Create an AI data assistant containing the baseline domain experience for your defined roles. Luckily, this is actually relatively easy, given the vast knowledge that LLMs already contain. It just requires writing a biographical sketch for the data assistance and feeding it to them so they know ‘who’ they are.
- Onboarding involves teaching the AI data assistant about your organization and what you expect of it. At a high level, this is analogous to onboarding a human. Much the same, you give them an array of organization-specific content so they can learn. For those who are more technical, I am not referring to training or fine-tuning the LLM but rather supplying documents as part of a Retrieval-Augmented Generation (RAG) approach.
- Test the AI data assistant to make sure it understands and can do a good job. Again, this is remarkably similar to what we do with humans. After we onboard and give them 30-60-90-day plans to ramp up, we test how they are doing and give them guidance and correction. The effectiveness of this with human employees depends on the manager. The same is true with AI data assistants, except it is a partnership between the manager and the AI data assistant maintainer.
A Few Less Abstract Use Case Examples
Here are a few anecdotal examples from my consulting practice.
Catalog Adoption Issues
I am continuously approached by data leaders who are trying to solve the puzzle of catalog adoption. The latest was a short time ago when the CIO of a large distribution and logistics company asked me about this. He has made a very substantial investment in a data governance program with a focus on capturing and curating data assets that his business stakeholders said they need. The only problem is that his team is having problems getting users to adopt and use the catalog.
Looking at the role classifications above, it’s clear that they have a beautiful reference library, but no one likes the librarian. This is a perfect use case for “hiring” a librarian AI data assistant that is super friendly and easy to work with. All users need to do is type a narrative conversation. Best of all, the reference library (catalog) investment already exists. All they need to do is onboard the new librarian.
Analytics Style Guide
A client, the chief operating officer of a national retailer, was frustrated that the charts and graphs used in the meetings he attends use inconsistent data representations. Sometimes, his managers do not even choose the best and most appropriate representation for the data they are presenting. This is causing a lot of wasted time, internal confusion, and difficulty translating cross-function performance and making decisions. He requested that we create a style guide and include best practices as part of the planned analytics learning and development program.
Including this as part of a data literacy program is a good idea, but what’s even better is to “hire” an analytics instructor who can coach people as they create their spreadsheets and presentations. The AI data assistant instructor would understand the organization-specific styles as well as best practices and could be asked for advice before managing, sharing, and presenting.
Finding the Experts and Answering Questions
I was engaged to take over analytics program management for a mid-sized multi-line insurance company. They were going through a major claims and policy management migration and trying to migrate their data warehouse and analytic reporting platforms simultaneously. One of the biggest issues they faced was the time people were wasting trying to figure out who the experts were on numerous old systems, new systems, and the new authoritative source of analytic data. Employees in almost all departments were routing email messages every day, playing ping-pong, searching for the best experts to help them and answer questions.
This was before LLMs, but in today’s world, it would be a perfect scenario for hiring a combo librarian and analyst AI data assistant. The librarian could point to specific system owners and top experts. Better yet, its analyst capabilities could be used to answer data questions and eliminate the need to ask others in the first place.
Can You Afford Not To?
We all know that it’s still early days with generative AI, and it’s far from perfect, but much like the early days of the internet or, more recently, the cloud, it’s a core capability that is not going away and will only improve.
The question is, would you rather have more help rather than less with your manpower-hungry programs? Also, would you rather have something that can be adopted virally or keep trying your current approach to drive data & analytics deep into company culture?
Where to Start
Most people want to start with technology. I love technology, but I’ve purposely ignored it here because I think starting with need and value is more important.
Here is my recommendation:
- Start by documenting the personas of your key stakeholders and internal data and analytic consumers. In those writeups, pay special attention to their learning, reference, and analytic needs.
- Create your job matrix as described above, with the explicit goal of defining job descriptions and definitions as if you were going to go out and hire.
- Start comparing your job needs against the capabilities of gen AI-based data assistants.
- Pick one job and create a pilot AI data assistant, and you will be out of the world of the hypothetical and on the path of learning and iterative improvement.
You will find that you have a latent and untapped pool of labor in the form of AI data assistants.