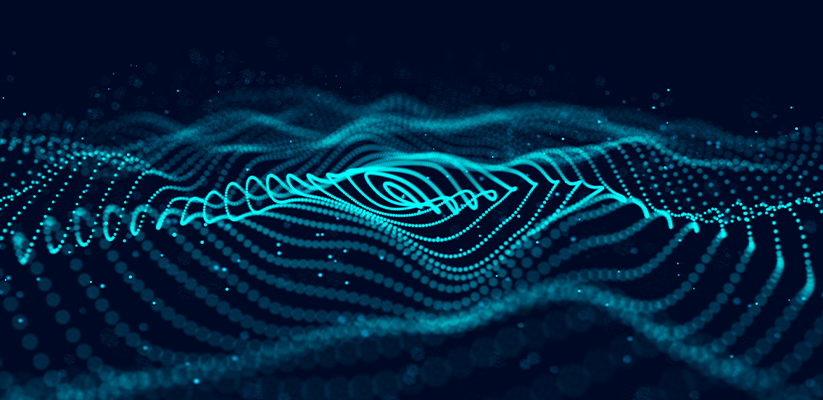
The data landscape has become more complex, as organizations recognize the need to leverage data and analytics for a competitive edge. Companies are collecting traditional structured data as well as text, machine-generated data, semistructured data, geospatial data, and more. Many organizations are transitioning to cloud platforms to harness the full potential of advanced analytics, including machine learning and natural language processing, and to accommodate intricate, diverse new analytical demands.
In this environment organizations face a number of challenges. In a recent TDWI survey, over 70% of respondents stated that they were struggling to get to the next stage of analytics maturity. There are many factors involved. One is that data is increasingly stored in multiple silos, making it hard to access all of the needed data for analytics. Silos also make it hard to get to a trusted version of the truth due to discrepancies between different systems that house the same data. Additionally, enterprises face a lack of alignment between parts of their businesses—usually between business and IT. A poor data or analytics strategy may impact analytics. Finally, the need for improved data literacy is a top priority among those we survey.
If organizations need timely access to diverse data to support new use cases made possible through advanced analytics, the question is whether or not they need a new approach to data, analytics, and people.
This is where data mesh comes in.
What Is Data Mesh?
Data mesh was first introduced in 2019 by Zhamak Dheghani. She said that data mesh is not an architecture per se but a sociotechnical paradigm that recognizes the interactions between people and the technical architecture and solutions in complex organizations. In other words, data mesh is a framework or a way of thinking. It arose because of all the complexities mentioned above. Data mesh has four key pillars:
- Domain-oriented ownership. Business domains own their own data, so ownership is decentralized. This idea is that that kind of ownership should scale out data sharing, and that domains should share data across organizational boundaries.
- Data as a product. Enterprises should make data accessible to those who need it and data should be viewed as a product. This means that the customers of data products should be satisfied with them, and the company should be organized to support a product view of data. Business domains are responsible for data quality, understandability, and interoperability.
- Self-service. In a data mesh, a self-service platform is used to empower teams. Multiple personas can make use of a self-service platform. This would lower costs and enable the development of not only dashboards, but also specific products such as network management for telecommunications providers, and a wide variety of other data applications.
- Federated governance. In a data mesh, governance is based on a federated model with team members from different business units sharing the effort. The data mesh model balances the autonomy of the domains with the required compliance, interoperability, and security of the mesh.
CDOs that TDWI interviewed say they support a number of data mesh principles and are already practicing them. For example, they believe in coordinated and federated governance. They like the idea of “data products.” Many of them are moving to a self-service model.
Opportunities and Challenges
In a 2022 TDWI survey, we asked respondents about data mesh, their plans for it, and opportunities and challenges. Respondents noted that data mesh should make it easier and faster for the business to access data. They said that data literacy can improve because if the data is in the domains and is a product businesspeople get involved with, a data mesh can increase literacy. Data products should be more effective, too, if domains own the data. Finally, if data is a product that is shared with customers, and all domains share their data, then theoretically all units will participate, getting away from silos.
However, respondents noted many challenges with data mesh. This included the fact that to many, the concept was esoteric and hard to understand. Many thought that domains wouldn’t share their data. They were concerned that the data would be hard to govern and worried about the impact data mesh would have on jobs. Respondents were also concerned about cost.
Data mesh does have many good principles: Data should be provided as a high-quality product with metadata. Self-service analytics should be enabled. Business domains should be involved in the data and the governance of that data. However, organizations are still concerned about how data mesh could actually work in practice.
Getting Started with Data Mesh
Before you begin, consider the following:
- Weigh the costs against the potential benefits. Organizations need to weigh the challenges against the benefits of the data mesh approach. For instance, moving from a centralized model to a decentralized one may make sense for organizations with multiple business units, where benefits might outweigh the costs, and they have the resources to staff each domain. It can be difficult for others.
- Consider new roles. Each domain must think about data as a product, which is another big change that will require a data product manager. This is a new role that will be needed for each domain, together with other roles that may not already be available in each domain, such as a data engineer or a data scientist. The data product manager is responsible for the data as well as how it is transformed and enriched; this person would also be responsible for identifying and assessing opportunities for gaining value from data. The data product manager is an important role and should be considered at the start of the data mesh journey.
- Evaluate the needed skills. Additionally, each domain will need its own data team to provide sharing, publishing, discoverability, and interoperability of all data assets within the mesh. Domains will need data management staff, software developers, and data analysts and data scientists to deliver the value in the data. Someone (possibly the data product manager) must define the KPIs and metrics to help track progress and measure success.
- Assess organizational models. The company must be organized to execute. Although there will be domains, the scope of those domains will need to be determined. Additionally, some organizations won’t have all the skills needed in their domain. Models such as a squad model may be used to deploy a squad to a certain domain when specific expertise is needed. There are also cross-domain functions that will need to be addressed. The data office is emerging as a new organizational construct; it is responsible for developing and delivering a business data strategy that meets the company’s needs, including leading the effort to develop data policies, standards, and governance; deriving value from data; and building a data-driven culture.
- Formal change management may be needed. All of this will, no doubt, involve power dynamics as the organization shifts from a centralized to a decentralized model. These power dynamics will need to be managed. How will the organization motivate teams to change and follow the data mesh principles? For instance, will domains just become data silos, hoarding their own data?
- Consider the architecture. Aside from the organizational considerations, companies will also need to determine how to implement a data mesh. One architecture that is emerging to support data mesh is a data fabric approach. The term “data fabric” has been used to describe a way to bring together disparate data in an intelligent fashion. Data fabric maps and connects relevant application data stores with metadata to describe data assets and their relationships. One approach to data fabric design uses data virtualization, a method that integrates heterogeneous and distributed data across multiple platforms without replicating it. The approach creates a single “virtual” data layer that unifies data and supports multiple applications and users. Data virtualization can create logical views in which the data looks consolidated, although the data has not been moved or physically altered. In a recent TDWI survey, nearly 50% of respondents had either implemented a data fabric approach or were planning to do so.
For more information on data mesh, come to Denodo Datafest AMERICAS in Boston, MA on September 12-14, 2023, where I’ll be participating in a panel on this topic alongside other thought leaders and industry experts.