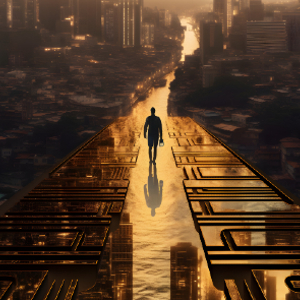
Harnessing the power of data has become critical in today’s digital age when information is abundant and decision-making is critical in many aspects of business. Understanding your data may unearth hidden insights and move your business ahead, whether you’re a small startup or an established enterprise.
However, going on the road of data analytics may appear daunting at first. Not to worry! We’ll walk you through the five steps that will turn your raw data into actionable insight.
1. Collect and Prepare Data
The first stage in any data analytics journey is to collect and prepare data. It entails obtaining essential information from numerous internal and external sources and verifying its quality and correctness.
- Determine the Data: To begin with, you need a clear understanding of what data you require to answer your key business questions. This could involve mining your existing databases, integrating data from different systems, or even sourcing it externally through APIs or web scraping.
- Clean the Data: Once you have identified the necessary data, it’s time to clean and transform it into a usable format. Data cleaning involves removing inconsistencies, duplicates, outliers, or any other anomalies that may hinder accurate analysis. Transforming the data includes structuring it in a way that aligns with your analytical goals — this could mean aggregating values, standardizing units of measurement or creating calculated fields.
- Prepare the Data: Data preparation also entails dealing with missing values by either inputting them using statistical techniques or making informed decisions about their impact on subsequent analyses.
During this stage, ensuring data security and compliance is critical. Implementing security measures like encryption techniques, user authentication, and access restrictions will protect sensitive data while meeting legal standards.
2. Data Exploration and Governance
Following data collection and preparation, data exploration and governance is the next stage in your data analytics journey. This phase focuses on delving deep into your data to identify hidden patterns, trends, and insights. It’s like going on a thrilling treasure hunt where every piece of information has the potential to provide vital information.
At this point, you must ensure that your data is clean, correct, and appropriately classified. Setting up solid governance systems is critical for keeping high-quality datasets that can be relied on for analysis. You may reduce mistakes and inconsistencies while also assuring compliance with relevant requirements by employing efficient data governance processes.
Exploring the immense ocean of available data necessitates a keen mind and sophisticated analytical tools. To explore relationships in your dataset, you’ll utilize a variety of approaches, including:
- Visualizations
- Statistical analysis
- Clustering algorithms
- Correlation matrices
This procedure assists you in gaining a better knowledge of the underlying issues that influence your company’s outcomes. Furthermore, it is critical to include stakeholders from other departments or teams during this stage to acquire multiple opinions. By using collective intelligence, collaboration improves problem-solving capacity.
3. Data Analysis and Modeling
The stage of data analysis and modeling is when all the acquired and prepared data comes to life. Here, you explore deeply into your data, looking for patterns, correlations, and insights that might help you make decisions. This level necessitates knowledge of statistical techniques and tools to examine the data efficiently.
Most Recommended Techniques
- Regression analysis
- Clustering algorithms
- Predictive modeling
Results Using Data Instead of Guesswork
- Pattern discovery
- Make forecasts
- Gain increased knowledge about your industry, sector, or company
- Identify growth possibilities
- Manage business operations
- Reduce risks and hazards within the business
- Pinpoint consumer preferences
- Improve supply chain operations
Modeling extends the process by constructing mathematical representations of real-world circumstances. Simulations enable organizations to test various tactics online before adopting them in the real world. This technique gives essential insight into possible outcomes and aids in the refinement of decision-making processes.
However, keep in mind that data analysis is not a precise science — it takes careful interpretation and consideration of the numerous aspects at play. Furthermore, as technology evolves and AI capabilities improve daily, including machine learning algorithms in the modeling process improves accuracy even more.
4. Data/AI/Machine Learning Enhanced Decision-Making and Planning
Businesses now have access to a variety of data that can be used for decision-making and planning thanks to technological improvements. Furthermore, with the incorporation of Artificial Intelligence (AI) and Machine Learning (ML), this process has grown in strength.
AI systems are capable of processing massive volumes of data at breakneck speed, discovering patterns and trends that people may miss. This allows firms to make better-informed decisions based on correct data.
Machine learning takes it a step further by continually refining its models via experience, allowing it to adapt and learn from new data without the need for explicit programming. It enables firms to automate complicated processes, optimize operations, and more accurately forecast results.
Businesses may minimize human error while increasing efficiency by integrating AI and ML technology for decision-making and planning operations. Companies can use these technologies to evaluate historical data, identify significant variables influencing their goals or objectives, and provide forecasts or suggestions based on these results.
Furthermore, AI-powered analytics may deliver real-time insights that can assist firms in staying ahead of the competition in today’s fast-paced business scene. They can immediately spot variations from expected outcomes by continually monitoring key performance indicators (KPIs) with machine learning techniques.
5. Monitoring and Evaluating
Monitoring and interpreting data are critical steps in the data analytics process. After you’ve collected and examined your data, it’s critical to keep track of its performance and evaluate the success of your strategy.
During this stage, you must define KPIs that are aligned with your company objectives. These KPIs will assist you in tracking development and measuring success. Setting realistic goals that can be easily tracked over time is critical.
You should use tools and technology that enable real-time tracking and reporting to monitor your data properly. This will help you rapidly detect any concerns or patterns that demand immediate action.
It is also critical to evaluate the findings of your data analysis. You may learn a lot about the success of your decision-making process by comparing actual results to predicted results. This review identifies areas for improvement and guides future planning.
Regularly evaluating and analyzing your data supports continual process optimization, discovery of growth prospects, and risk avoidance. It enables continual learning from previous experiences, resulting in more informed decision-making in the future.
Remember that monitoring and evaluation are continuous processes that occur as firms change over time. Adopting these stages in your data analytics journey will equip you with the actionable insights required for long-term success.
The Data Analytics Journey Can Bring a Lot of Information Into Focus
Any firm may benefit from embarking on a data analytics journey that is both thrilling and revolutionary. Businesses may obtain important insights, make educated choices, and enhance their operations by using the power of data.
The five steps of your data analytics journey are interrelated components that build a unified framework for extracting meaningful insights from your organization’s massive quantity of data. Each phase builds on the preceding one until your judgments are motivated by accurate forecasts rather than simple intuition.